AIDA: explAinable multImodal Deep learning for personAlized oncology
In the fourth industrial revolution we are witnessing a fast and widespread adoption of artificial intelligence (AI) in our life, healthcare included. Advancements in deep learning (DL) should make significant contributions in this area supporting diagnosis, prognosis and treatment decisions. Most of the DL models consider only unimodal data, neglecting information available in other modalities of patient digital phenotypes, e.g. histopathology images and electronic health records (EHRs). As interpreting imaging findings is multimodal by its very nature, AI needs to be able to interpret such modalities together to progress towards higher informative clinical decision making.
AIDA tackles this challenge advancing multimodal DL (MDL), an area of great interest at its infancy. It studies how deep neural networks (DNNs) can learn shared representations between different modalities by investigating when to fuse the different modalities and how to embed in the training any process able to learn more powerful data representations. AIDA also searches for an optimal MDL fusion architecture, robust to missing modalities or missing data, and it studies multimodal regularization to improve stability, algorithmic speed-up and to reduce overfitting. It also considers approaches mitigating training from scratch, even when datasets of reduced size are available as it happens in healthcare.
A key impediment to the use of DL-based systems in practice is their black-box nature that does not permit to directly explain the decisions taken. Explainable AI (XAI) is now attempting to improve trust and transparency, but its investigation in healthcare is in an early stage. AIDA explores XAI methods for MDL using attention mechanisms, generating textual and fine-grained visual explanations, creating and testing human-understandable concepts, providing counterfactual examples and enabling the interaction between the algorithm and the final user. This can have a disruptive impact since model opacity makes it difficult for doctors and regulators to trust it.
Further to offer AI researchers a framework for MDL yielding a richer and trustworthy data representation producing much improved performance compared to use a single modality, AIDA tackles the association between radiomic, pathomic and EHRs in precision oncology to predict the patient outcomes in terms of progression free survival, overall survival, relapse time and response rate in non-small cell lung cancer, representing the 85% of all lung cancer cases. This represents one of the first attempts to integrate all such modalities as the literature still overlooks potential useful information they gather. AIDA performs a prospective study in two hospitals, experimentally evaluating the developed framework even in comparison with conventional clinical markers. This application in precision oncology strengthens the social and economic project impact, also aligned with the pillars of the Horizon Europe program.
Objectives
By interfacing several areas of AI, AIDA pursues the following general objectives: i) to investigate methods for MDL, studying when and how to learn shared representations, comparing with UDL; ii) to investigate methods to explain decisions taken by multimodal DNNs, exploiting attention mechanisms, textual and fine-grained visual explanations, counterfactual explanation and human-understandable concepts; iii) to deploy the breakthrough nature of MDL in personalized oncology, fusing radiomic, pathomic and EHRs data to predict treatment responses.
These general objectives introduce also AIDA counterpart goals. They are: i) to perform a prospective study with patients affected by NSCLC, i.e. one of the major killer, since a publicly available repository with such multimodal data does not exist; ii) to experimentally validate the MDL methods developed on such prospective data and also on retrospective data already available in the PI’s unit, comparing also with conventional clinical markers.
Who we are
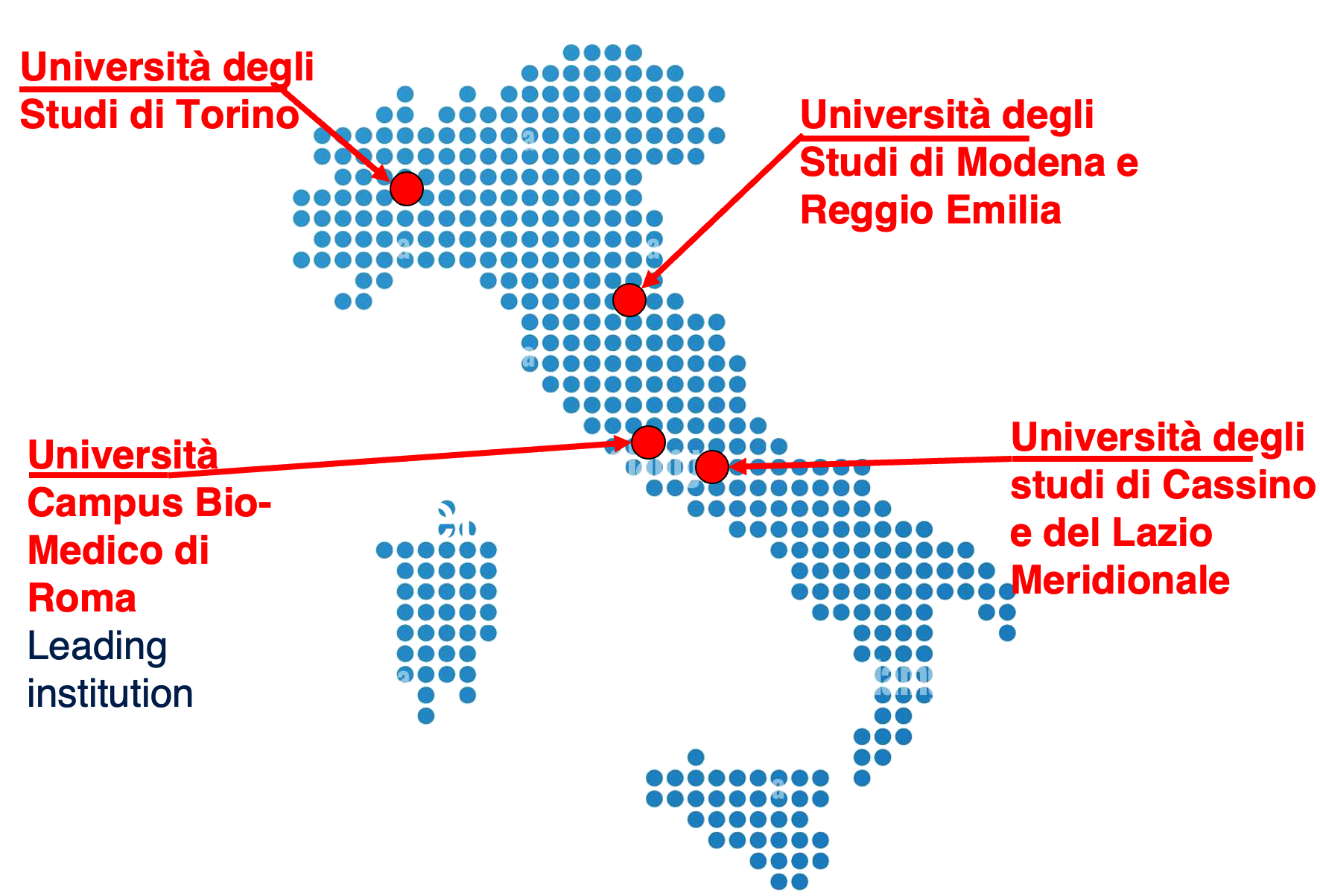
Visual abstract
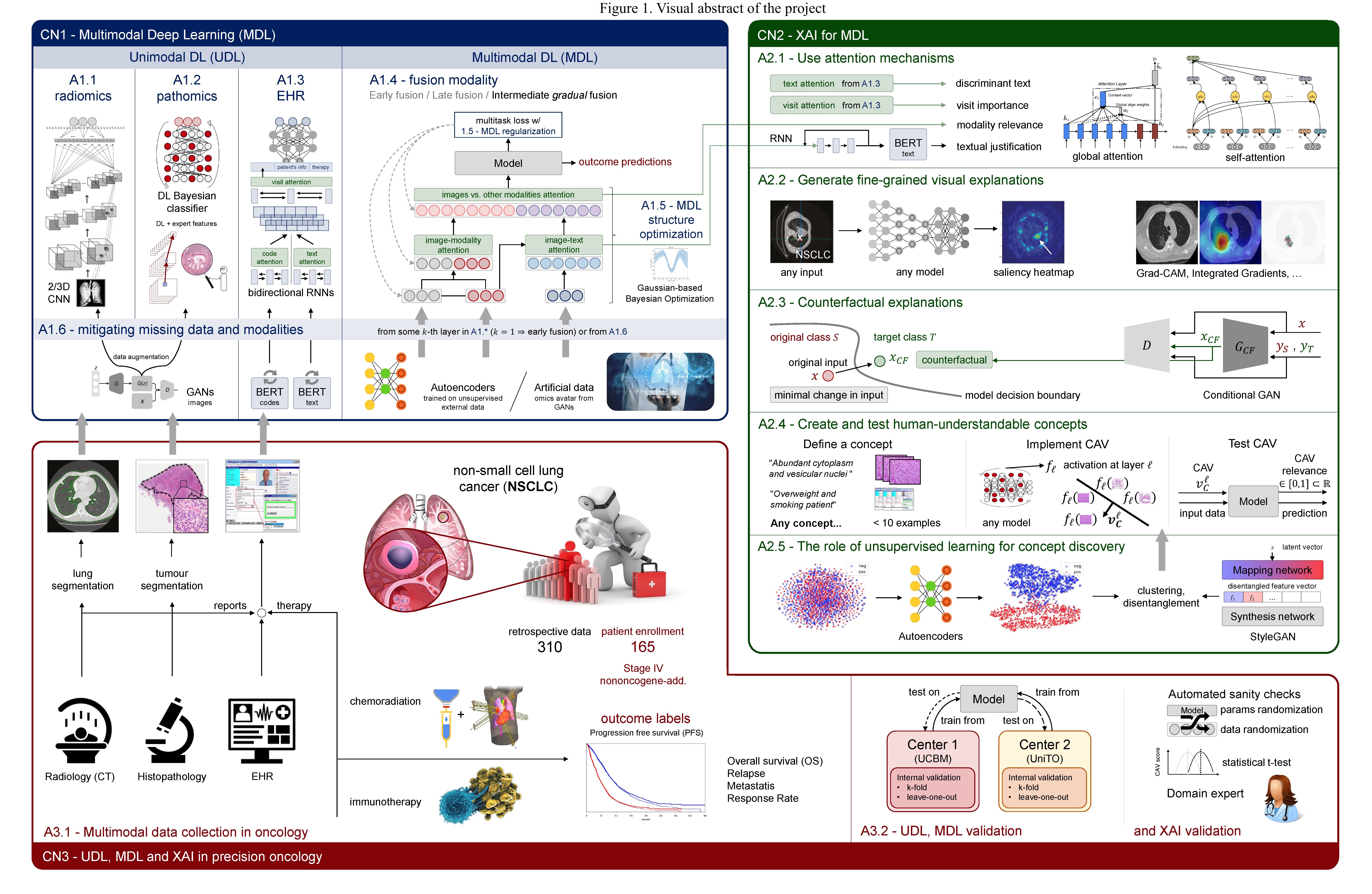